A breadth of knowledge
MSE students displayed a wide range of research topics at the 2024 Research & Industry Showcase.
By: Theresa Gabrielli
Photos: Matt Hagen/University of Washington
Top image: Attendees of the 2024 Research & Industry Showcase crowd around posters to hear about the latest research from current MSE students.
Materials science and engineering plays a role in virtually every engineering and scientific field. Nowhere is that more apparent than at the UW MSE Research & Industry Showcase. Over 120 students, faculty, staff, alumni and members of industry attended to hear from 23 students representing nearly a dozen different research groups at the event on April 1, 2024.
This showcase is more than just an opportunity for students to practice giving research talks in front of an audience of their peers. It is also a chance for capstone mentors and department alumni to visit, reconnect with former professors, and celebrate the deep connection between the department and the many industries that students are hired into after graduation.
Here, three students share how their research is making impacts across the spectrum of topics under the MSE umbrella.
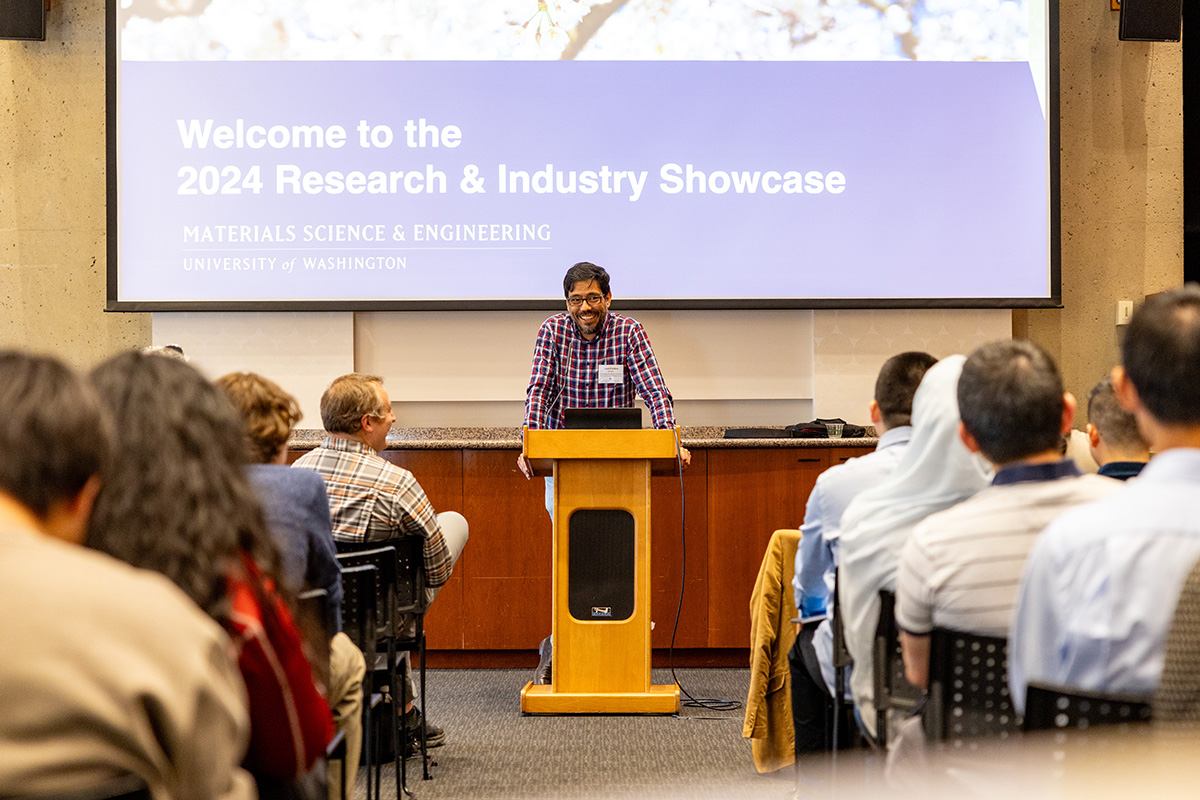
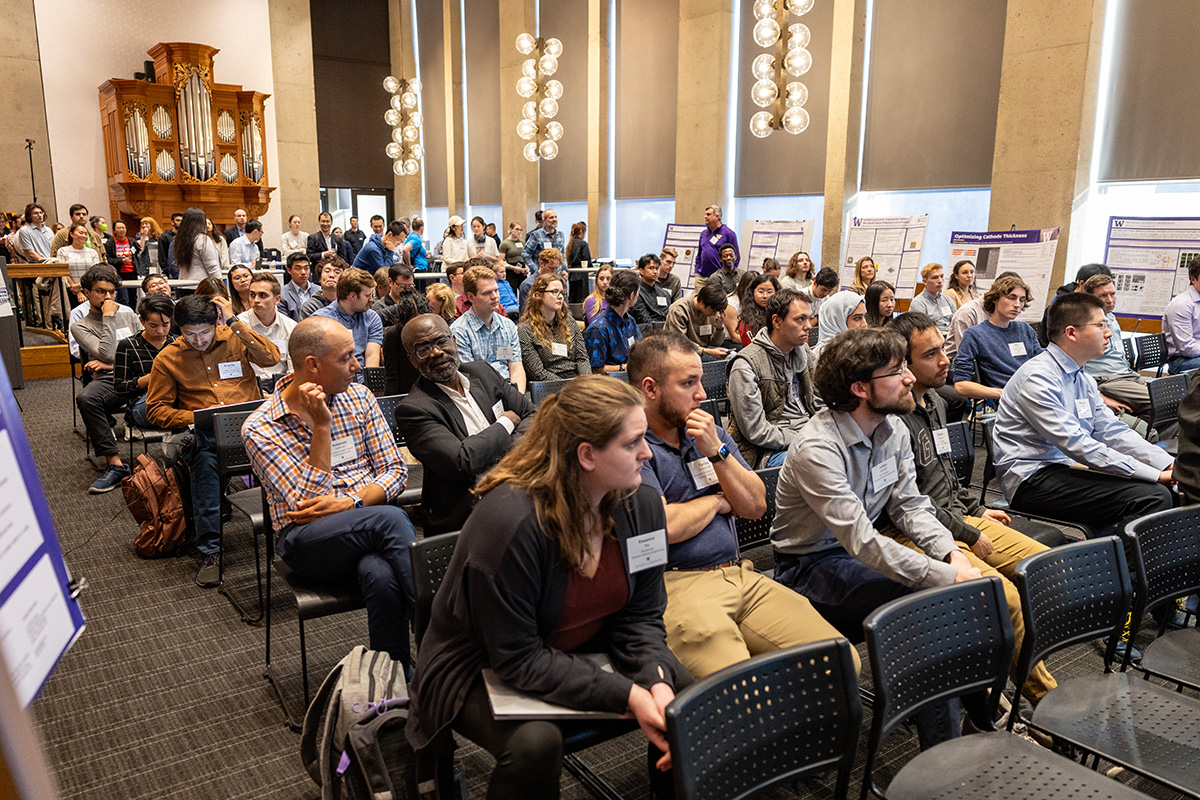
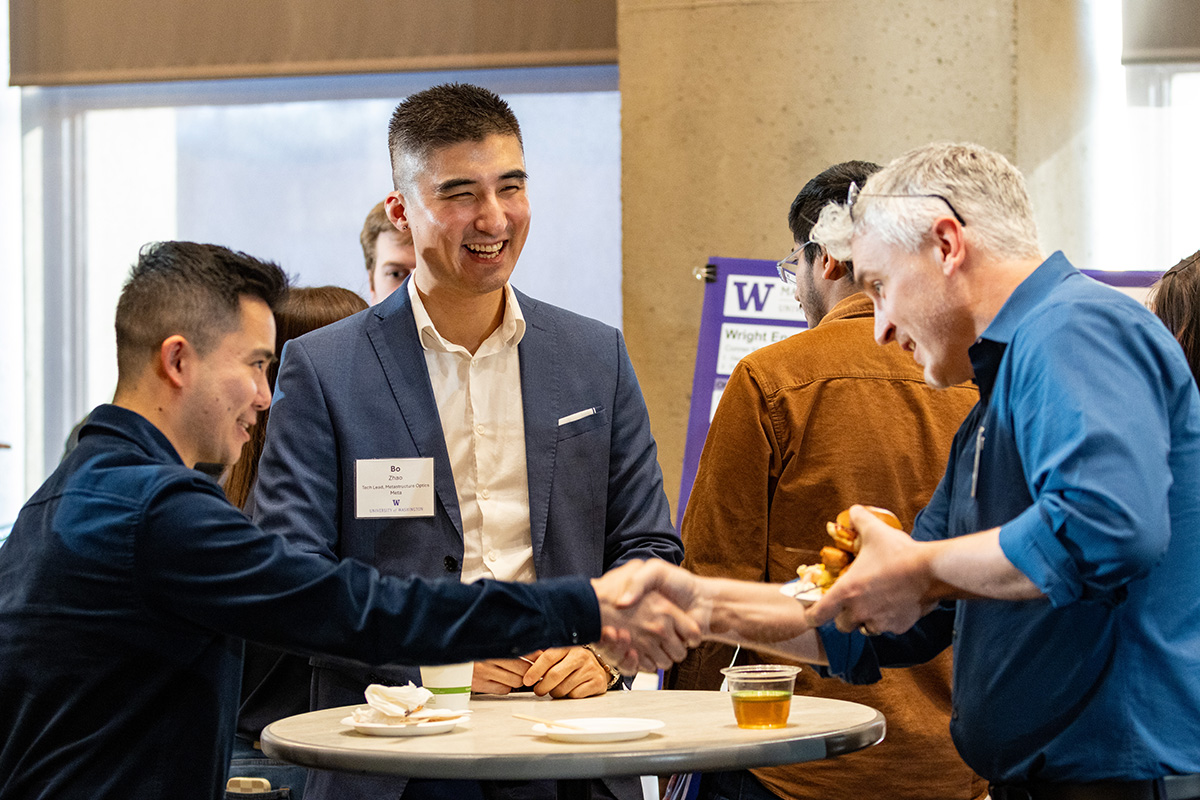
Left: Professor Juan Carlos Idrobo served as emcee for the event. Top right: Showcase attendees listen to graduate student oral presentations. Bottom right: Professor Peter Pauzauskie greets two former students.
The path toward knowledge doesn’t always run straight
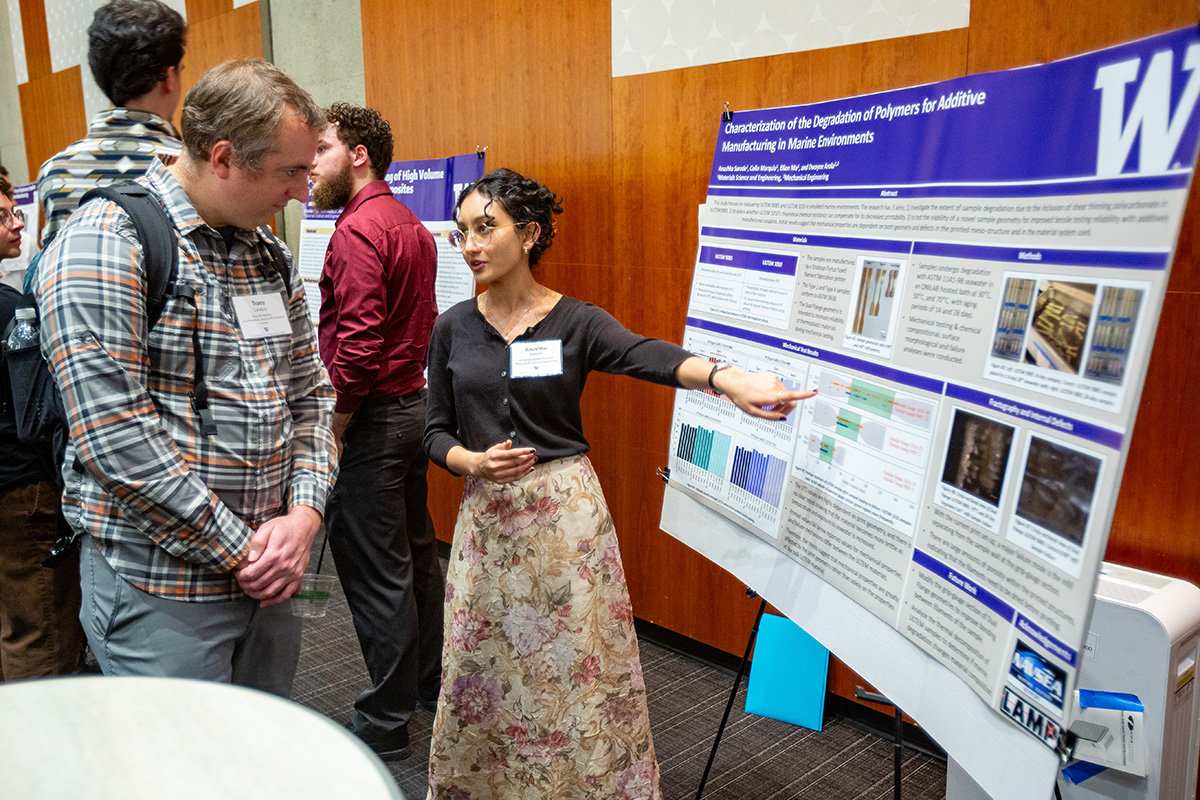
Senior Anushka Sarode had intended to determine how two thermoplastic materials would degrade in seawater. One is strong but known for creating defects during 3D printing, while the other is a blend with added polycarbonate to improve the printing process but could weaken its resistance to seawater. Sarode and her team in the Arola lab wanted to test a third variable, however: how does the shape of the sample impact the materials’ reliability?
Sarode tested three styles of printed samples – two with standard, well-known dimensions, and one that was significantly shorter and thicker, called the “Dual Flange” geometry. The team hypothesized that this sturdier-looking geometry would be better suited for mechanical testing of materials that have been additively manufactured.
The results showed samples failing in unexpected ways. A magnification of their cross sections revealed the issue – during printing and mechanical testing, the interior of the Dual Flange samples had separated from the sample wall, creating large gaps that left them vulnerable. The printing method will need to be adjusted before the team can attempt the experiment again.
“There’s a lot of work for improvement, but I’m excited because it’s an achievable goal,” she said.
Finding a cure
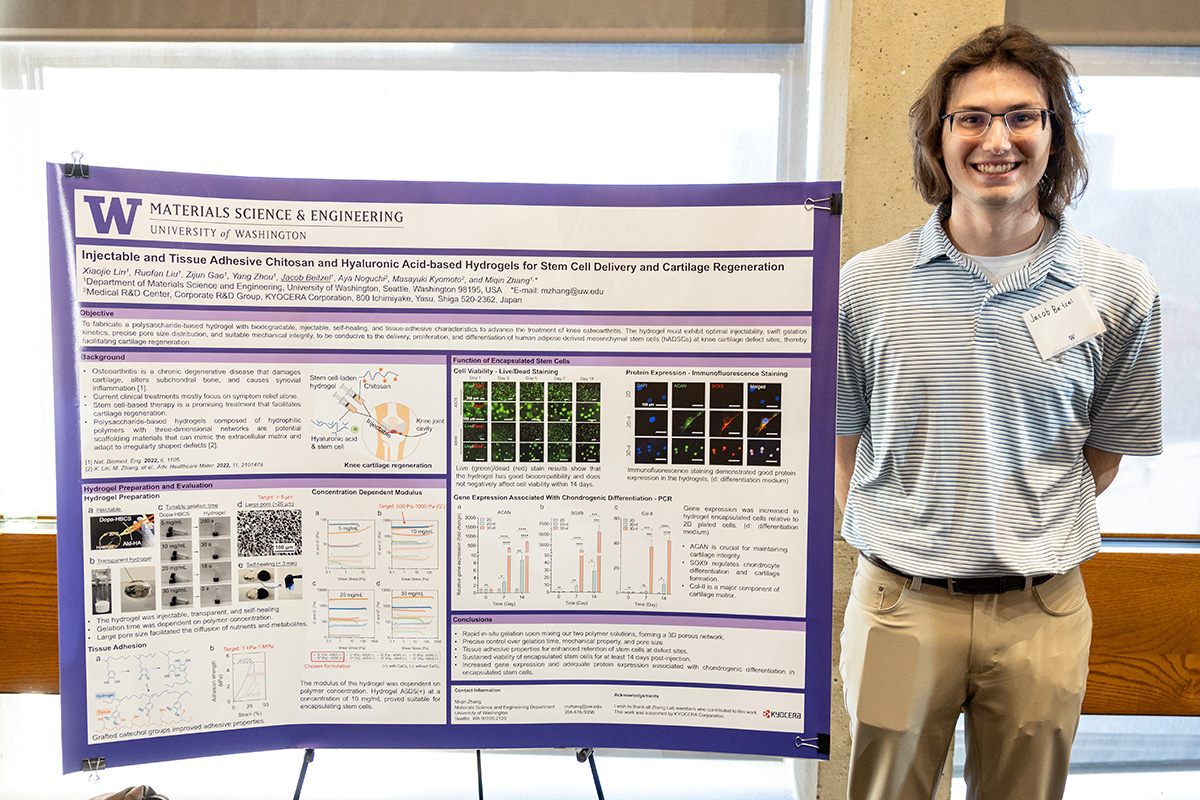
Osteoarthritis is a painful degenerative disease that damages the cartilage and bones around joints, and for which treatment mainly consists of managing symptoms. Master’s student Jacob Beitzel and his peers, under the guidance of Xiaojie Lin and Prof. Miqin Zhang, may have a solution: an injectable hydrogel containing stem cells that could reverse cartilage loss, effectively curing the condition.
The hydrogel they’ve developed is a mixture of two polymers, chitosan and hyaluronic acid, which chemically bond to form a gelatinous, 3D porous network. After testing various formulas, they created a hydrogel with the desired gelation time, pore size, tissue adhesion and other critical factors. Testing also revealed that their optimal hydrogel could maintain a significantly higher proportion of viable stem cells for an extended duration compared to current therapies. “The hydrogel keeps the stem cells encapsulated and at the treatment site,” said Beitzel. Those stem cells can then turn into cartilage cells and rebuild the joint. “Making a gel that has all of our targeted properties is very exciting,” Beitzel added.
Improving efficiency with AI
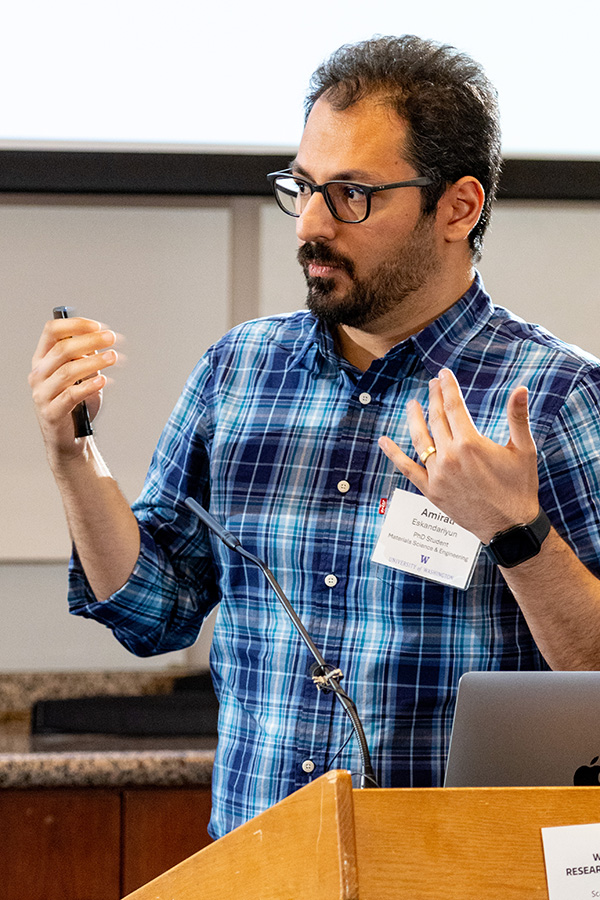
When developing new composite materials for use in aerospace, the amount of physical testing necessary can be immense. Every new material in every new shape intended for every new purpose has to run the gamut of process, environmental and strength tests. What’s more, these tests often destroy the material sample, leading to enormous amounts of material waste that cannot be recycled.
Second year Ph.D. candidate Amirali Eskandariyun is working on a machine learning tool capable of generating simulations to identify where and how a composite sample will fail. These simulations could be used to weed out the less-ideal candidates rapidly and without consuming any materials. As with anything involving AI, the tool can’t work without an expansive dataset. Eskandariyun developed both low-fidelity and high-fidelity simulations in a multiscale multiphysics framework capable of producing large datasets in a short amount of time.
“This will be faster and less expensive than conducting [physical] tests over and over,” Eskandariyun said. “It would also be possible to find the best combinations of materials, like carbon fiber and resin, before running full tests.”
Originally published April 11, 2024.